AI Sentiment Analysis and Classification is a pivotal area within artificial intelligence, focusing on the interpretation and categorization of human emotions and opinions expressed in textual data. By leveraging Natural Language Processing (NLP) techniques, AI systems can discern the sentiment—positive, negative, or neutral—behind written content, enabling businesses and organizations to gain valuable insights into public perception, customer feedback, and social media discourse.
Understanding Sentiment Analysis
Sentiment analysis, also known as opinion mining or emotion AI, involves the systematic identification, extraction, and study of affective states and subjective information from textual data. This process enables AI systems to comprehend the emotional tone behind words, facilitating a deeper understanding of human sentiments.
Applications of Sentiment Analysis
-
Customer Feedback Analysis: Organizations utilize sentiment analysis to process customer reviews, surveys, and social media comments, allowing them to gauge public opinion and enhance products or services accordingly.
-
Market Research: By analysing consumer sentiments, businesses can identify market trends, assess brand reputation, and make informed strategic decisions.
-
Political Analysis: Sentiment analysis aids in understanding public opinion on political issues, candidates, and policies, providing valuable insights for campaign strategies.
3. Techniques in Sentiment Analysis
-
Lexicon-Based Approaches: These methods rely on predefined lists of words associated with specific sentiments. While straightforward, they may struggle with context and sarcasm.
-
Machine Learning Approaches: By training algorithms on labelled datasets, these methods can learn to classify sentiments based on patterns in the data.
-
Deep Learning Approaches: Utilizing neural networks, particularly Recurrent neural networks (RNNs) and Long Short-Term Memory (LSTM) networks, these models capture complex patterns and contextual information in text.
4. Challenges in Sentiment Analysis
-
Sarcasm and Irony Detection: Identifying sarcasm and irony remains a significant challenge, as these expressions often convey sentiments opposite to their literal meaning.
-
Contextual Understanding: Words can have different meanings based on context, making it essential for AI systems to comprehend the surrounding text to accurately determine sentiment.
-
Multilingual Analysis: Processing sentiments across different languages and dialects requires models to understand linguistic nuances and cultural contexts.
5. Future Directions
The field is evolving towards more sophisticated models that integrate multimodal data, such as combining text, audio, and visual inputs, to enhance sentiment analysis accuracy. Additionally, advancements in transformer architectures, like BERT and GPT, are pushing the boundaries of understanding and generating human-like text, offering new opportunities for sentiment analysis applications.
In summary, AI Sentiment Analysis and Classification is a dynamic and rapidly advancing field, offering profound insights into human emotions and opinions. As AI technologies continue to evolve, their ability to understand and interpret human sentiment will become increasingly refined, opening new avenues for applications across various sectors.
Recent Developments in AI Sentiment Analysis

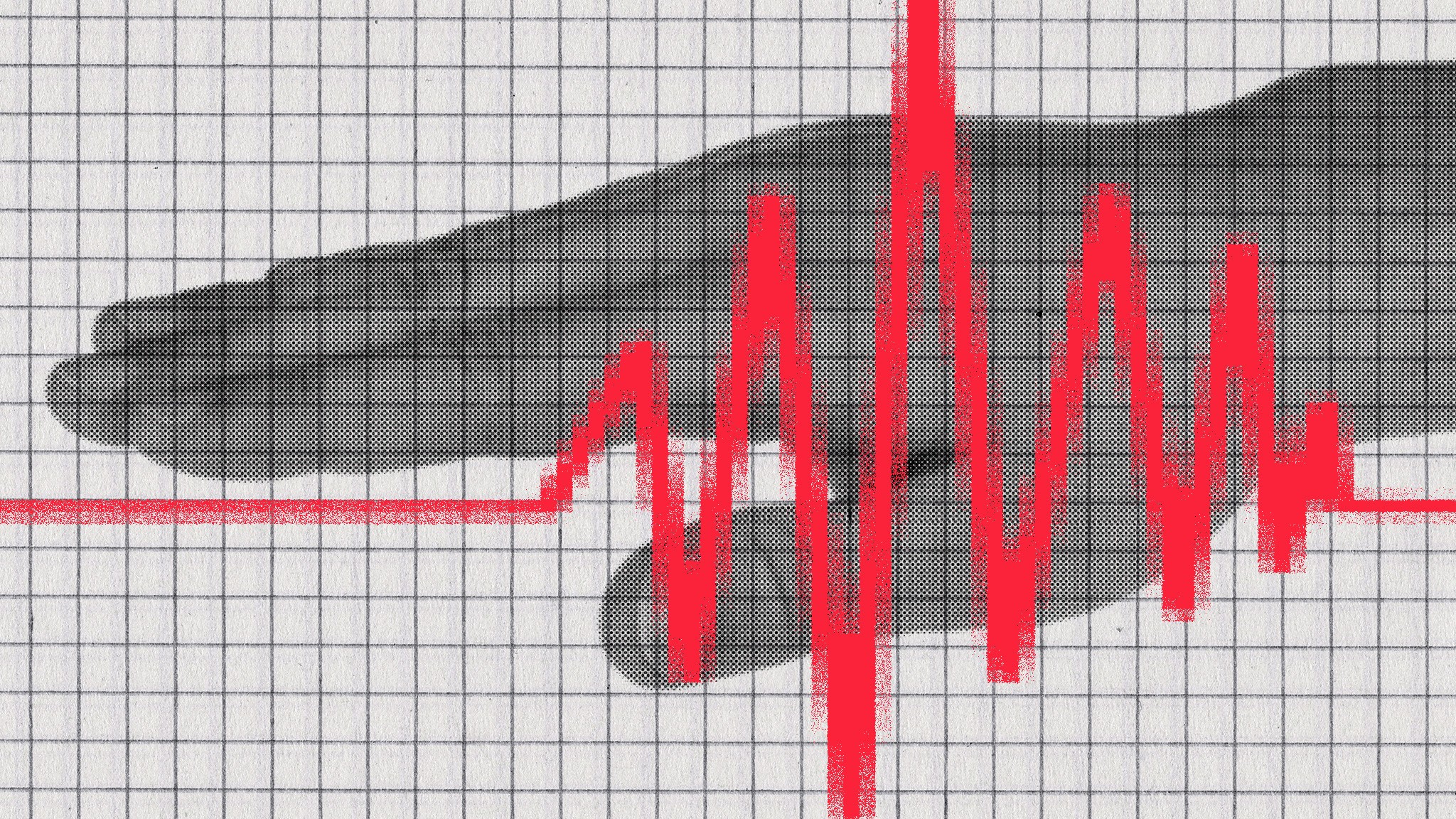

0 Comments